🔑 In 10 minutes you will learn:
- The 4 core values of Datumo
- How Datumo tries to maintain the core values
- Different types of data validation and their downsides
- Datumo’s unique algorithms/systems to acquire quality data
AI is only as smart as the quality of the training data.
This article is based on a speech given by David Kim, founder of DATUMO.
AI models achieve intelligence from the manually annotated data. We call the annotated data “training data.”
AI models and training data are deeply implemented in our daily lives. For instance, automated vehicles are trained with manually annotated data to detect and recognize objects.
Now it’s all about Data-centric AI.
The trend has changed from focusing on AI models to focusing on quality datasets.
-From model-centric to Data-centric AI
What is “quality data”?
David points out four main characteristics of quality data.
As shown in the image above, data must be annotated accurately, as intended. The essence is to not have any data that violate the guideline. Since all data are annotated manually, it is important to educate the annotators and have strict validation system in order to achieve data accuracy.
The key is to have guidelines with high readability.
Since the beginning of foundation, Datumo had a dedicated <User Guidelines Team> that solely focuses on writing easy and clear guidelines for the annotators.
Easy and clear guidelines enhance the work efficiency.
“Set accurate guidelines → Train crowd-workers → Achieve high quality data” is an unchanging truth.
Mandatory exams in order to participate in data annotation
One of Datumo’s methods for quality control is the exam system. All crowd-workers are required to pass complex exams based on guidelines in order to participate in data annotation.
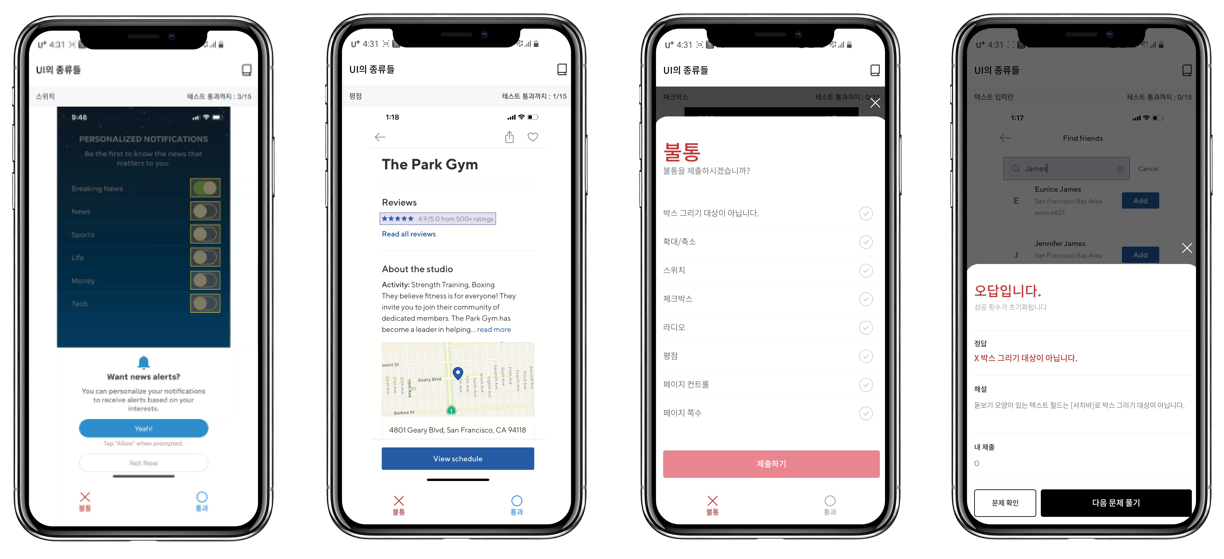
Strict validation based on Datumo’s experience & algorithm
Experienced crowd-workers and in-house inspectors cross-validate the annotated dataset to filter inaccurate data. Furthermore, Datumo is the only platform in Korea to run strict validation based on the reliability of the inspectors.
Single inspection
→ Highly dependent on the accuracy and attentiveness of the inspector
Cross-validation based on majority rule
→ Inspectors of low accuracy may outnumber those of high accuracy and thus, spoil data validation
Data validation based on inspector reliability inference algorithm is more accurate than single inspection or cross-validation based on majority rule.
Datumo is the only one to carry out validation based on inspector reliability.
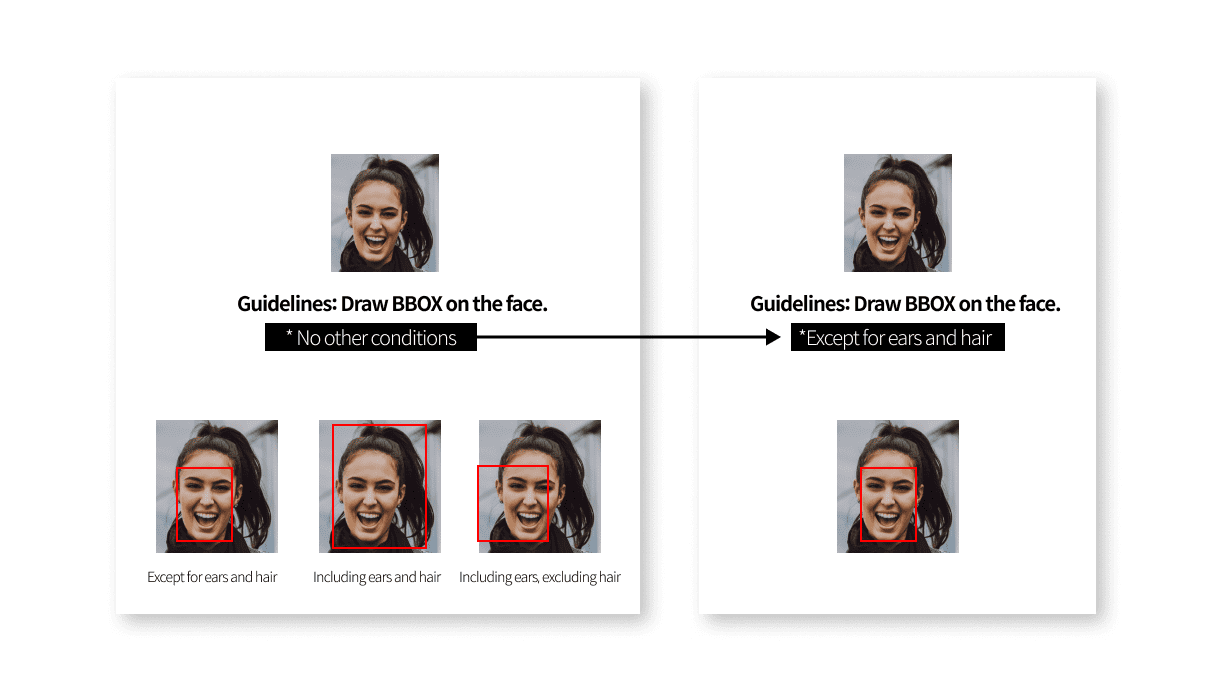
Objective labeling rules to maintain consistency
Datumo focuses on achieving consistent data by making sure the guidelines leave no room for ambiguity. For instance, when collecting or labeling dataset related to speech or emotion, we try to set standards in our guidelines so that everything falls under a certain category in order to avoid subjective interpretations depending on the annotator.
What technology does Datumo use in order to maintain consistency?
One of the most common issues in bounding box datasets is different box sizes depending on the annotator. As shown in the image above, without a specific guideline, Box 1 and Box 2 could be either correct of wrong depending on the inspector’s perspective. In order to avoid such situation, Datumo came up with a solution: an inner guiding box(patented). An inner guiding box, which is Datumo’s own-developed UI system, provides standards for both drawing and validating bounding boxes around the target object.
Dataset that covers a variety of cases that AI would face
It is important to expand the “coverage” of datasets by considering the environment of collecting data when planning the whole process.
Data collecting process based on collecting environment
The essence of expanding coverage is to provide AI models with a variety of cases that AI could face in the real world.
For facial image datasets, Datumo collected 1,100 crowd-workers through our own developed crowd-sourcing platform Cash Mission and hypothesized 3 different lightings, 8 different situations, and 11 different angles, which resulted in 264 different cases.
Redundant data filtering system to ensure data variety
Similar, redundant data reduce the value of the whole dataset. Due to its quantity, it is almost impossible to manually filter such data. This is why we came up with our own data filtering system based on machine learning. As the only ones to implement such system, Datumo developed an AI model that automatically detects three image data that look similar to the newly submitted image. Then, the inspector manually validates whether the newly submitted image is redundant or not.
Good dataset must be unbiased. It is important to have a well-balanced dataset, consisting of elements weighting similarly to each other.
Avoid bias by various classification
For a text data collecting project regarding automobile issue reports, we wanted to avoid ending up with just a few most common issues reported repeatedly. Thus, we specified the types of issues into five different categories- inoperable/visual/auditory/tactile/olfactory – and made sure to collect similar amount of data for each category.
As a result, we were able to minimize data imbalance and provide our client with the variety of data they have asked for.
From Model-centric to Data-centric AI
Based on such effort and technology, Datumo strives to make “good data.”
Data quality was the core element of the business since foundation. We will continue to optimize and improve our technology and system to construct a smoother flywheel. In Data-centric perspective, consistent and high-quality data are of greatest value.
Datumo aims to drive impact in the AI industry by innovating the system of collecting AI training data, one of the worst bottlenecks in the industry, through revolutionary technology.