In the rapidly advancing field of artificial intelligence (AI), Large Language Models (LLMs) have transformed various industries by offering powerful text processing capabilities. However, while these pre-trained models are impressive, they are not always suitable for specific industry applications without additional customization. This is where LLM fine-tuning comes into play, particularly when focusing on LLM fine-tuning datasets and effective LLM fine-tuning methods.
Fine-tuning LLMs enables companies to customize these models to meet the precise needs of their industries. From healthcare to finance, and legal to retail, the importance of fine-tuning LLMs using industry-relevant datasets cannot be overstated. In this blog, we will delve into how fine-tuning works, why fine-tuning methods are essential, and how the selection of the right fine-tuning datasets can transform your business.
The Role of LLM Fine-Tuning Datasets in Customizing Models
The quality and relevance of the LLM fine-tuning datasets used during the customization process are critical to achieving successful results. These datasets should be industry-specific and task-focused to ensure the model can accurately perform specialized functions.
For instance, in healthcare, using a general corpus might lead to irrelevant or misleading outputs. But when fine-tuned with high-quality medical datasets, the model can learn to understand medical terminologies, drug interactions, and patient records, significantly improving its diagnostic and advisory capabilities.
Real-World Example:
Google Health and other healthcare institutions are known to use large medical datasets for LLM fine-tuning to improve their models’ accuracy in diagnosing diseases. Fine-tuning models on relevant data ensures that they can perform complex tasks, such as interpreting EHRs, with precision.
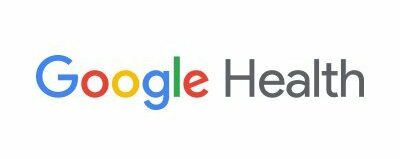
Essential LLM Fine-Tuning Methods for Industry-Specific Customization
The process of fine-tuning requires selecting the best LLM fine-tuning methods for optimizing model performance. Whether it’s supervised learning, reinforcement learning, or domain-adaptation methods, businesses must use strategies that align with their specific goals.
One common LLM fine-tuning method involves supervised learning, where the model is further trained using labeled datasets. For example, financial institutions fine-tune LLMs on datasets like earnings call transcripts and regulatory reports using this method to enhance their predictive accuracy and analysis capabilities.
Another key fine-tuning method is domain adaptation. This involves taking a general model and making it effective in a specific area, such as legal contract review, by training it on a relevant dataset. Domain adaptation helps the model understand the unique language of a particular field, ensuring better results in specialized applications.
Selecting the Right LLM Fine-Tuning Datasets for Your Industry
Choosing the appropriate LLM fine-tuning datasets is one of the most crucial steps in optimizing an LLM for a specific industry. These datasets should be as representative as possible of the language and tasks the model will perform. For example:
- In healthcare, clinical datasets and research papers are essential for fine-tuning models to assist in diagnostics.
- In finance, training on historical financial data and earnings reports will enhance the model’s ability to analyze trends and predict market movements.
- In the legal industry, using datasets comprising contract clauses and court rulings will significantly improve the model’s performance in legal document analysis.
Evaluating Your LLM with Industry-Specific Benchmarks
After applying the right LLM fine-tuning methods and datasets, it’s important to evaluate the model’s performance using industry-specific benchmarks. This ensures that the model not only works but excels in the chosen domain. Evaluation metrics should be relevant to the industry – for instance, accuracy in diagnosing diseases in healthcare or the correctness of legal clause identification in law.
LLM fine-tuning is more than just tweaking an AI model – it’s about customizing it to solve real-world problems in specific industries. The key to success lies in selecting the right LLM fine-tuning methods and leveraging domain-specific LLM fine-tuning datasets. By doing so, businesses can unlock the full potential of AI, ensuring that their models provide more accurate, valuable, and relevant solutions.
Incorporating these fine-tuning methods and carefully chosen datasets is crucial for building industry-specific AI solutions that deliver results. By focusing on LLM fine-tuning with targeted datasets, companies can achieve significant improvements in efficiency, accuracy, and overall business impact.