As artificial intelligence (AI) technologies continue to shape modern business, the role of robust data governance has grown essential. Effective data governance ensures the quality, consistency, and security of data, enhancing both the performance and reliability of AI models. Through comprehensive governance frameworks, organizations establish a solid foundation for data management, enabling AI-driven innovation, regulatory compliance, and strategic decision-making.
The Core of Data Governance: Master Data Management (MDM)
Master Data Management (MDM) is essential for effective data governance. MDM ensures that core business data—such as customer, product, and supplier information—is handled in a standardized and consistent way across the organization. By maintaining a “single source of truth,” MDM frameworks reduce data redundancies and improve accuracy. This approach benefits critical business functions and has a direct impact on AI model quality.
Example:
Consider Amazon’s recommendation system. By using a unified view of customer data, Amazon can personalize suggestions, enhancing the relevance and accuracy of its AI-driven recommendations. This unification is only possible when MDM ensures consistent and accurate customer data across multiple systems.
Organizations typically use one of three MDM models:
- Centralized: Ideal for organizations requiring strict data control, this model provides a single point of oversight, enhancing consistency and compliance.
- Federated: This model synchronizes data from multiple sources, allowing flexibility for large, diverse datasets.
- Co-existence: A hybrid of centralized control and distributed access, enabling data management across both legacy and modern systems—an adaptable approach in fast-evolving environments.
Key Components of a Data Governance Framework
A robust data governance framework encompasses several key elements to ensure data quality, accessibility, and compliance. Core pillars include:
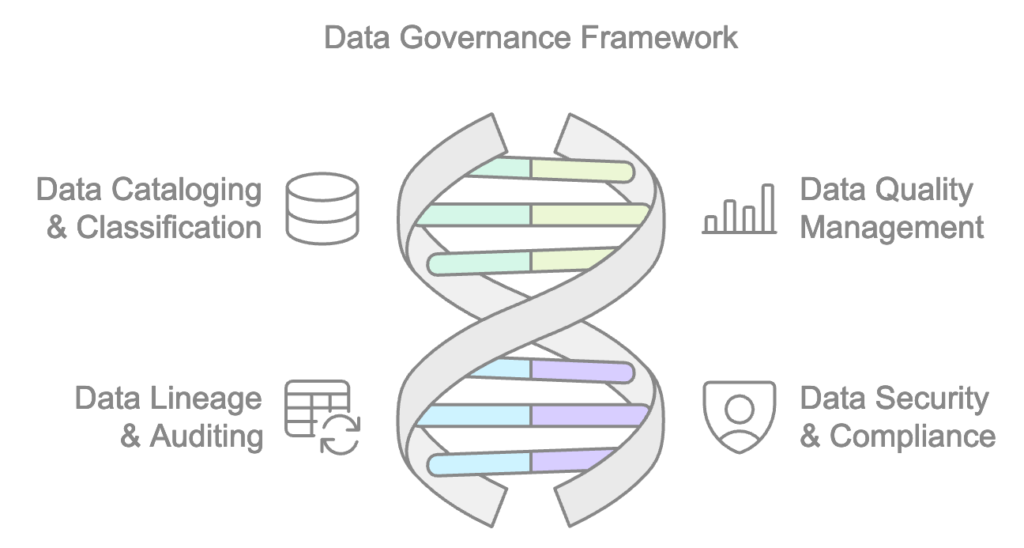
Data Cataloging and Classification: By organizing data into accessible categories, cataloging improves discovery and regulatory compliance. Centralized data catalogs support metadata and lineage management, essential for tracking data origins and transformations.
Data Quality Management: High-quality data is crucial for reliable AI outcomes. Attributes like accuracy, completeness, and timeliness are prioritized, with automated tools increasingly used to monitor quality, minimizing human error and providing AI with consistent datasets.
Data Lineage and Auditing: Tracking data’s lifecycle—from origin to transformation—enables organizations to manage compliance, conduct root cause analyses, and maintain AI model reliability.
Data Security and Compliance: Given regulations like GDPR and CCPA, frameworks must incorporate robust privacy and security measures, such as access control and data masking. Compliance checks help organizations adapt to evolving privacy laws and industry standards.
The Symbiosis of AI and Data Governance
Data governance forms the backbone of successful AI initiatives. It provides the quality and consistency that AI models need to deliver accurate results. MDM supports this by consolidating and harmonizing data from various sources. AI-driven solutions for fraud detection, predictive analytics, and customer engagement rely on data that remains both current and trustworthy.
To address AI’s specific requirements, data governance frameworks now accommodate unstructured data, such as images and audio, which are increasingly integrated into AI applications. Enhanced MDM solutions deliver AI-specific features, including automated data profiling, lineage tracking, and error detection. These capabilities streamline data preparation and elevate model outcomes.
Implementation: Challenges and Best Practices
Implementing data governance often faces challenges, from organizational silos to the need for real-time data access. To overcome these hurdles, companies can adopt the following best practices:
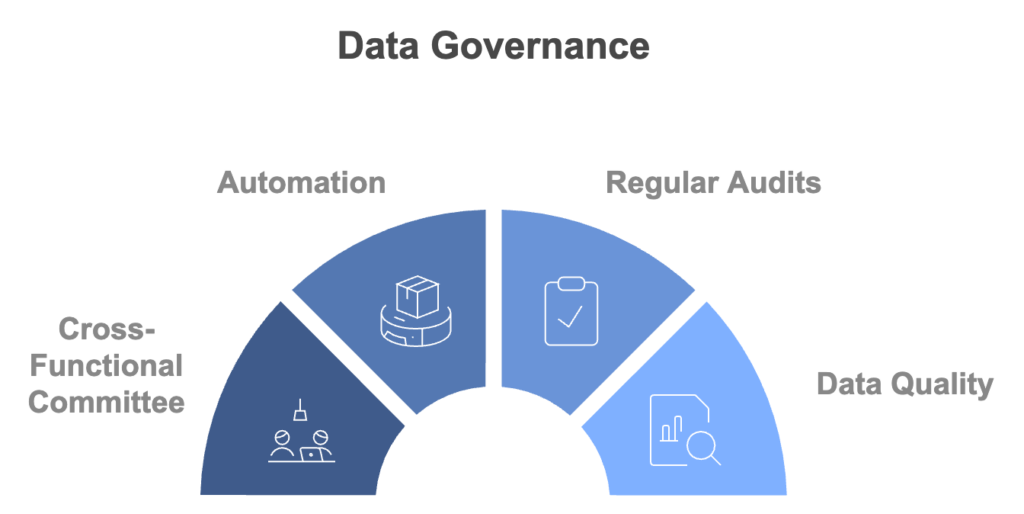
Establish a Cross-Functional Governance Committee: Data governance thrives on collaboration among data owners, executives, and stewards across departments. A governance committee ensures a cohesive strategy and consistent application of data policies.
Automate for Consistency: Automation in cataloging, quality checks, and auditing reduces manual intervention and supports consistency across the data lifecycle. Tools like Informatica and Collibra facilitate these processes, enhancing efficiency and reliability.
Conduct Regular Audits and Compliance Reviews: Routine audits ensure governance practices remain aligned with regulatory requirements. Compliance checks prepare organizations for changes in data privacy laws and industry-specific standards.
Prioritize Data Quality and Transparency: Investments in data quality management tools and transparent reporting practices help teams track improvements, identify issues, and refine policies for sustained governance effectiveness.
The Future
Data governance is no longer just a compliance necessity; it is integral to AI advancement and business transformation. As organizations continue to adopt AI, governance frameworks will need to evolve to handle diverse data types and increasing real-time requirements. Innovations in AI will likely influence governance strategies, particularly as tools that assess data bias, model transparency, and ethical considerations become more mainstream.
In today’s data-driven landscape, data governance is a strategic asset, essential for achieving reliable AI and informed decision-making. By establishing comprehensive governance frameworks with MDM at their core, organizations ensure that their data assets are accurate, secure, and accessible. Effective governance not only supports AI initiatives but also drives operational efficiency, reduces risk, and strengthens competitive advantage in an AI-centered business environment