On the 14th, Alibaba Group and its subsidiary Ant Group unveiled Animate-X, a model capable of transforming any image into video. Similar models have emerged in the past, with some even reaching commercial service levels. What unique features does this newly published paper offer?
Why Animate-X?
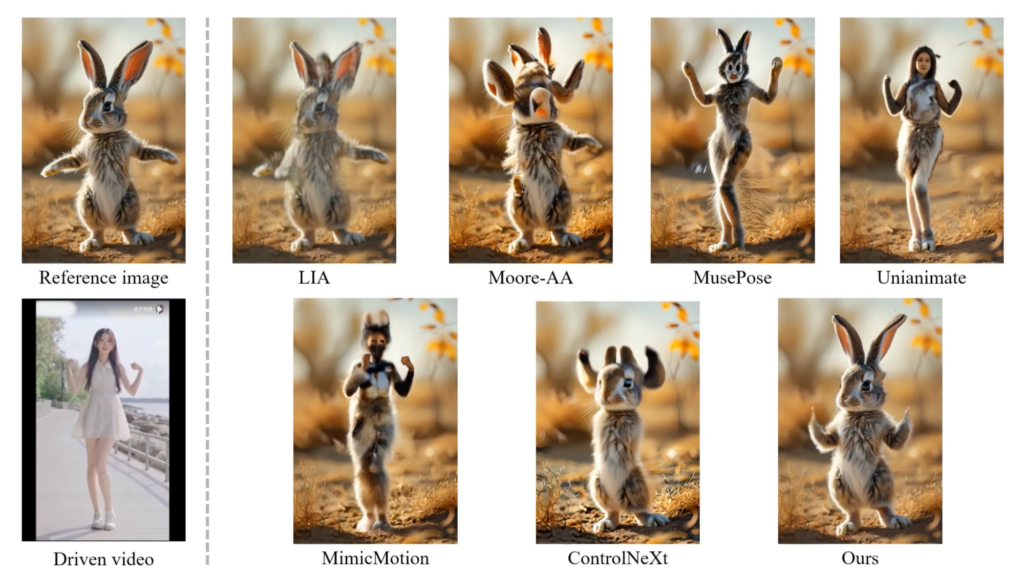
Make-A-Scene model that generates images based on sketches. Source: Meta AI Blog
How Does Animate-X Work?
To overcome the above issues, the researchers designed a Pose Indicator that can guide poses from both implicit and explicit perspectives. This approach adds detailed imagery and clarifies the complex interactions between shape and pose that the traditional skeleton method lacks. Let’s look at each concept more closely:
Implicit Pose Indicator (IPI)
: Using the CLIP model, IPI extracts features from the image. CLIP is a model trained to understand the relationship between images and text, going beyond visible features to interpret the concept or meaning that an image conveys. Trained to comprehend abstract contexts by linking text descriptions with images, CLIP enables IPI to capture underlying meanings and background information not attainable through a pose skeleton alone.
Explicit Pose Indicator (EPI)
: EPI addresses mismatched situations where the reference image and target pose differ significantly. For instance, if a short-armed rabbit needs to mimic a long-armed human pose, EPI adjusts these elements to bridge the gap. By simulating these mismatches, EPI fine-tunes specific pose elements to ensure a natural alignment between character and motion. Working alongside IPI, EPI supplements the concrete movements of a pose while capturing abstract scene contexts, producing more realistic and cohesive results that are otherwise difficult to achieve with just a pose skeleton.
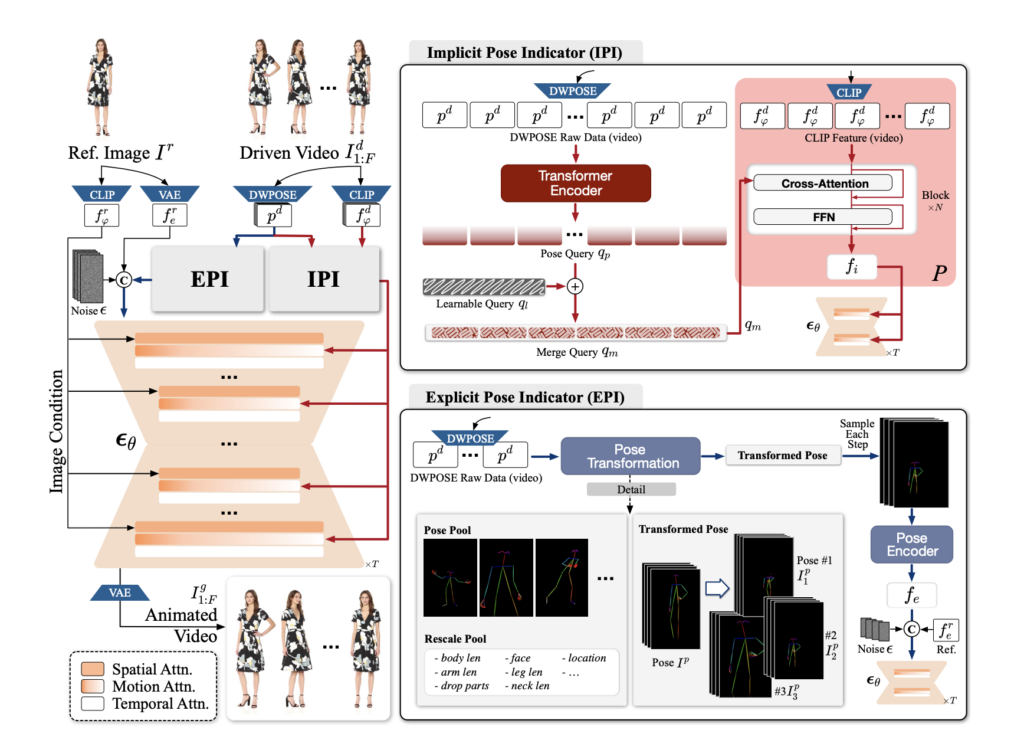
The Architecture of Animate-X.
Integrating IPI and EPI for Enhanced Motion Representation
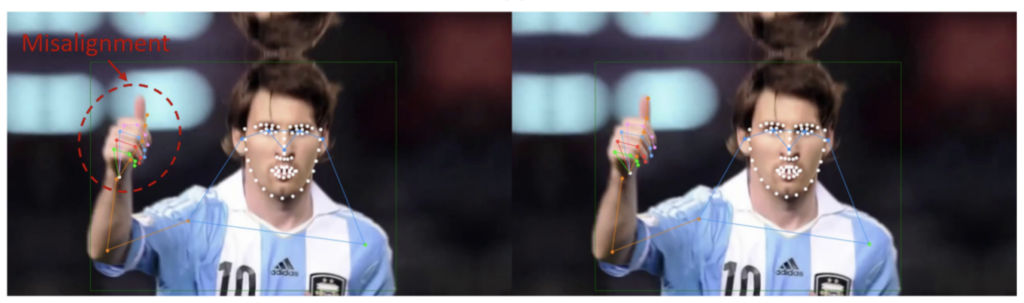
The result of using DWPose to adjust misalignment of fingers. Source:
The results are below. Despite none of the subjects in the video being actual humans, each character adopts poses naturally. Notably, even characters without hands or legs exhibit well-adapted poses that align with the traits of the reference image. This showcases the model’s ability to generalize pose characteristics effectively.
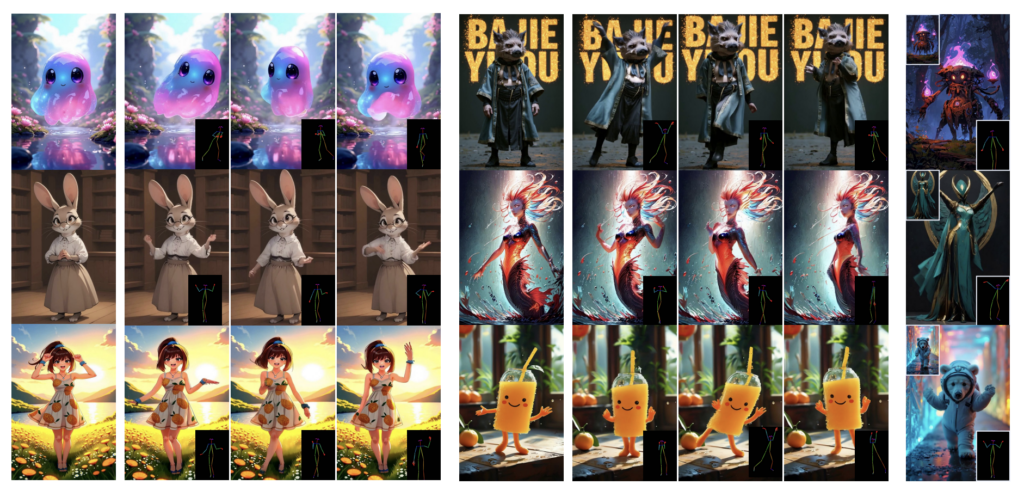
Additionally, the researchers introduced the Animated Anthropomorphic Benchmark (A² Bench), aimed at extending evaluation standards—previously focused on human poses—to include anthropomorphic animations. Animate-X achieved a much higher quality than existing models, setting a new state-of-the-art (SOTA) standard.
What Exactly Are IPI & EPI?
The core idea of this research lies in integrating explicit and implicit information into the generative model. In other words, it incorporates both human-understood concepts and model-learned concepts when generating images. The researchers conducted experiments to observe the results when only EPI or IPI was used. The outcomes are below. In the case without IPI, the model attempts to understand and mimic the movement, while in the case without EPI, the panda maintains its shape, albeit somewhat unnaturally.
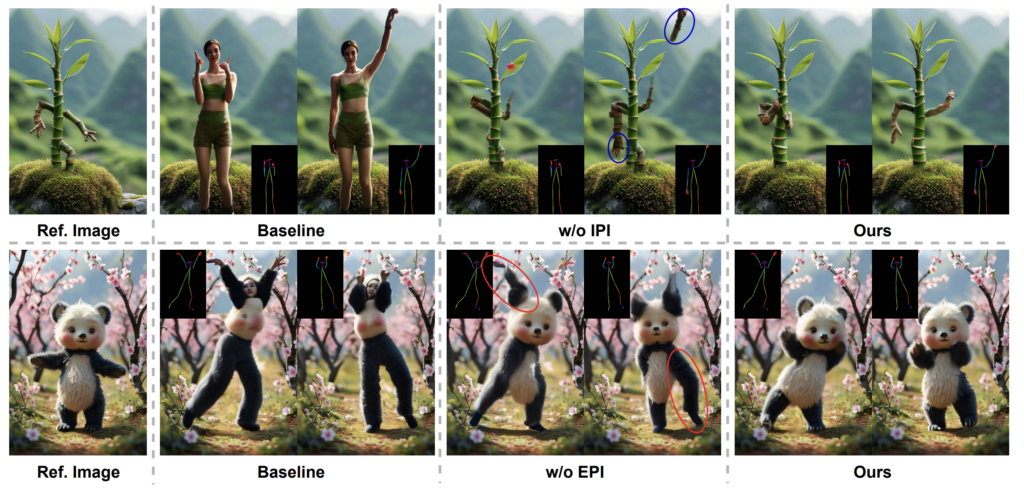
IPI preserves the external appearance and prevents the generation of unnatural outcomes (like a plant having human hands). EPI ensures that the reference subject and pose align accurately—such as keeping a panda’s ears correctly positioned. The final result is a harmonious combination of these two elements.
Animate-X pushes the boundaries of complex character animation generation. It offers an innovative approach that maintains consistency in identity and motion across diverse character types. By combining IPI and EPI, this new model structure achieves natural and flexible movement that goes beyond simple pose estimation. This advancement sets a promising new standard, not only for animation but also for applications in gaming, virtual reality, and digital content creation.