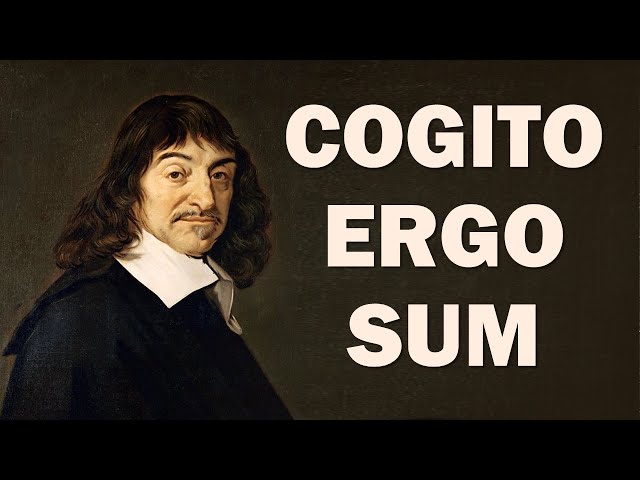
I think, therefore I am.
Descartes defined humans as thinking beings, grounding the very idea of existence in the capacity to think. Centuries later, we now face artificial intelligence systems that seem to think on their own.
One recent model has attracted attention for reportedly outperforming both Meta’s LLaMA 4 and DeepSeek’s R1. It was developed by Deep Cogito, an AI startup founded by former Google senior software engineer Drishan Arora. The company’s name, taken directly from Descartes’ philosophy, signals more than just branding—it reflects a clear and intentional vision.
Superintelligence: AI Beyond Human Intelligence
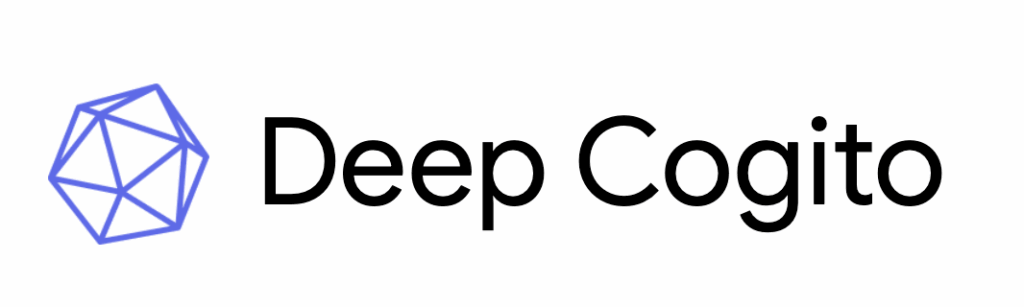
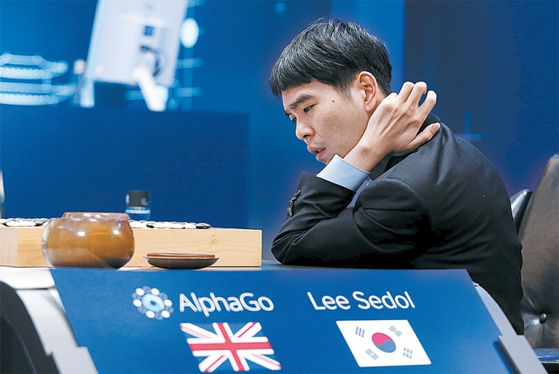
Lee Sedol playing against AlphaGo. Source: Google.
When AlphaGo faced Lee Sedol, many expected the human champion to dominate. In the end, Sedol won just one out of five games. Since then, AlphaGo and other game-playing AIs have shown that in narrow domains with clear rules, AI can not only match but surpass human performance.
Two key factors enabled this breakthrough:
Advanced reasoning: the use of massive compute to reach solutions well beyond traditional methods
Self-improvement: the ability to refine itself through iterative feedback, without constant human oversight
But AlphaGo’s knowledge stopped at Go. True superintelligence goes further—it can define new problems and solve them across different domains.
That’s what Deep Cogito aims to build: a general-purpose system that moves beyond specialized tasks. And early results suggest they’re on the right track. The company has released models ranging from 3B to 70B parameters, outperforming most open models, including LLaMA, DeepSeek, and Qwen, on a wide set of benchmarks. Notably, the 70B model even surpasses Meta’s 109B LLaMA 4 MoE in some areas.
So, what’s driving this performance?
Deep Cogito's Key Strategies
Deep Cogito trained its models using a method called Iterated Distillation and Amplification (IDA), which is a long-theorized strategy now realized in practice to help models move beyond domain-specific limits.
IDA follows a simple two-step cycle:
Amplification
The model is given more compute and prompted to perform complex reasoning, such as multi-step thinking, tool use, and other advanced tasks. This expands its capabilities beyond its current baseline.Distillation
The insights gained during amplification are distilled back into the model’s parameters. This lets the model handle similar problems more efficiently in the future, without repeating the full reasoning process.
With each cycle, the model improves. It becomes smarter over time, not through static data or manual tuning, but through its own repeated learning loop.
The most striking part? This doesn’t require human supervision. Unlike traditional training, which relies on human feedback or labeled datasets, IDA enables intelligence to scale through compute and algorithmic design alone. It’s a promising step toward truly autonomous, general-purpose superintelligence.
Results
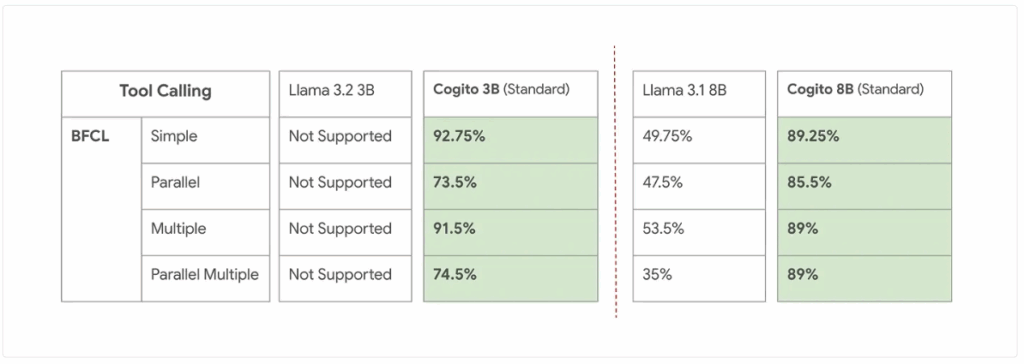
Tool Calling for smaller models. Source: Deep Cogito.