The Limitations and Necessity
How Graph RAG Works
Graph RAG goes beyond merely retrieving data—it divides and organizes it into meaningful units. Let’s take a closer look at how it works step by step.
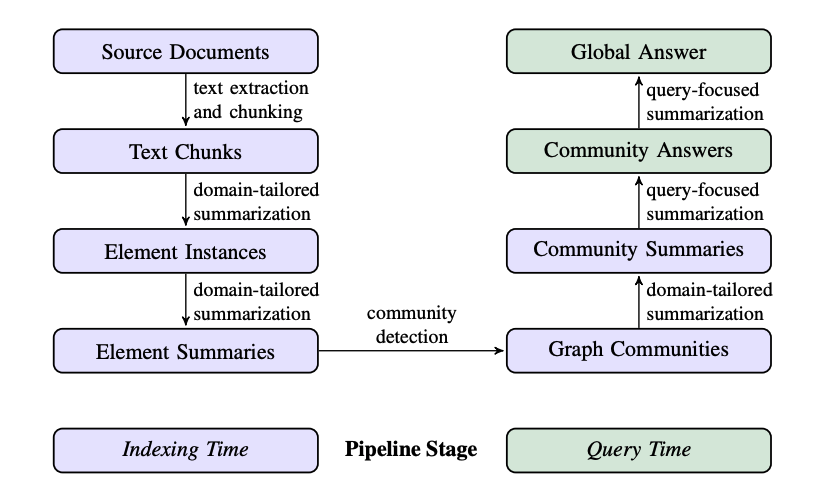
Graph RAG pipeline.
1. Data Splitting (Source Documents → Text Chunks)
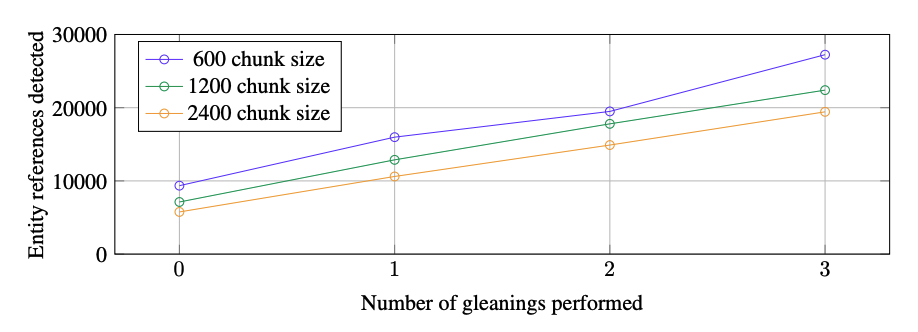
How the entity references detected varies with chunk size and gleanings.
2. Extracting Key Elements (Text Chunks → Element Instances)
- Nodes: Independent units of information, such as entities (e.g., people, places, or concepts)
- Edges: Connections or relationships between entities (e.g., “A is part of B”)
3. Generating Element Summaries (Element Instances → Element Summaries)
4. Creating Graphs and Communities (Element Summaries → Graph Communities)
5. Summarizing Communities (Graph Communities → Community Summaries)
Each community is summarized into a report using LLMs. These summaries include key nodes and edges and can function as:
- Indexes to answer specific questions.
- Standalone insights to understand the dataset’s structure and meaning even without a query.
- Leaf-Level Communities: Detailed summaries include key nodes, edges, and covariates, ordered by importance and added to the LLM’s context window.
- Higher-Level Communities: Summaries from leaf communities are aggregated. If context window limits are exceeded, lower-level summaries are compressed into shorter text while retaining critical information.
6. Generating Responses (Community Summaries → Community Answers → Global Answer)
Back into the Library
Imagine an LLM receiving the question, “What are the key trends in the tech industry today?” and generating a response using Graph RAG.
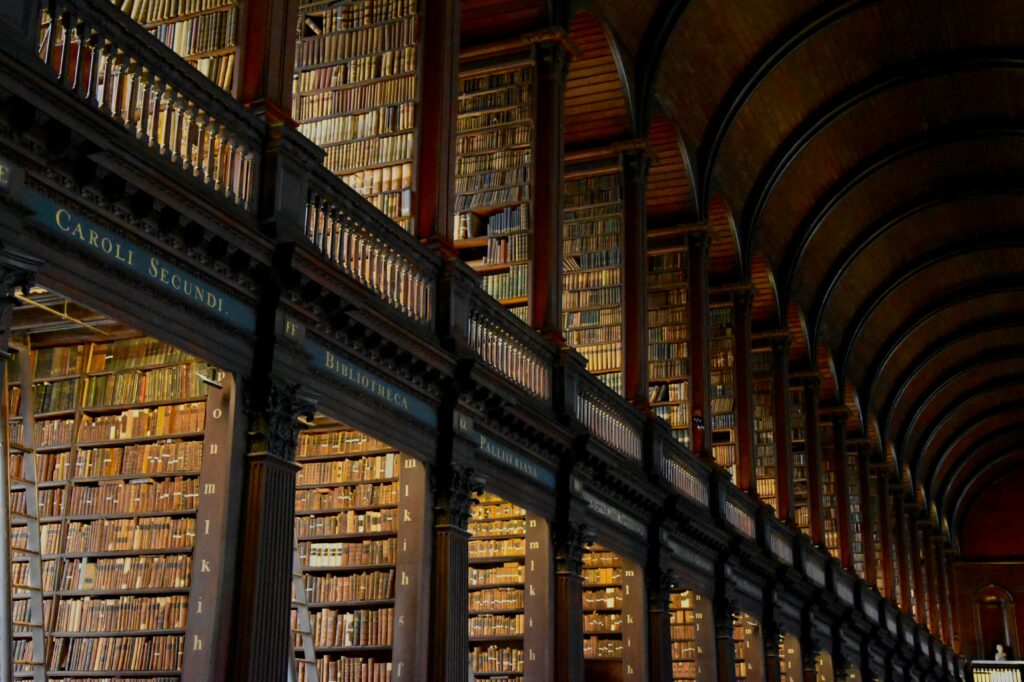
Photo taken by Guillaume Henrotte
Community Summarization:
The dataset is divided into multiple communities. For instance:
- An “AI Ethics” community might include nodes like “AI Transparency” and “Responsible Data Usage.”
Question Processing:
- For the question “What are the key trends in the tech industry today?”, it references summaries from the “AI Ethics,” “Data Privacy,” and “Generative AI Applications” communities.
Final Response Construction:
- AI Ethics Community: “AI transparency and responsible data usage are critical topics of discussion.”
- Data Privacy Community: “Data protection regulations are tightening, driving advancements in technologies for secure personal data management.”
- Generative AI Applications Community: “Generative AI is being applied across industries, including content creation, customer service, and product design.”
These responses are then combined to form a comprehensive and structured final answer:
“The key trends in the tech industry today are AI ethics, data privacy, and generative AI applications. AI transparency and responsible data usage are emphasized, while data protection regulations are being strengthened. Additionally, generative AI is expanding its applicability across various industries.”