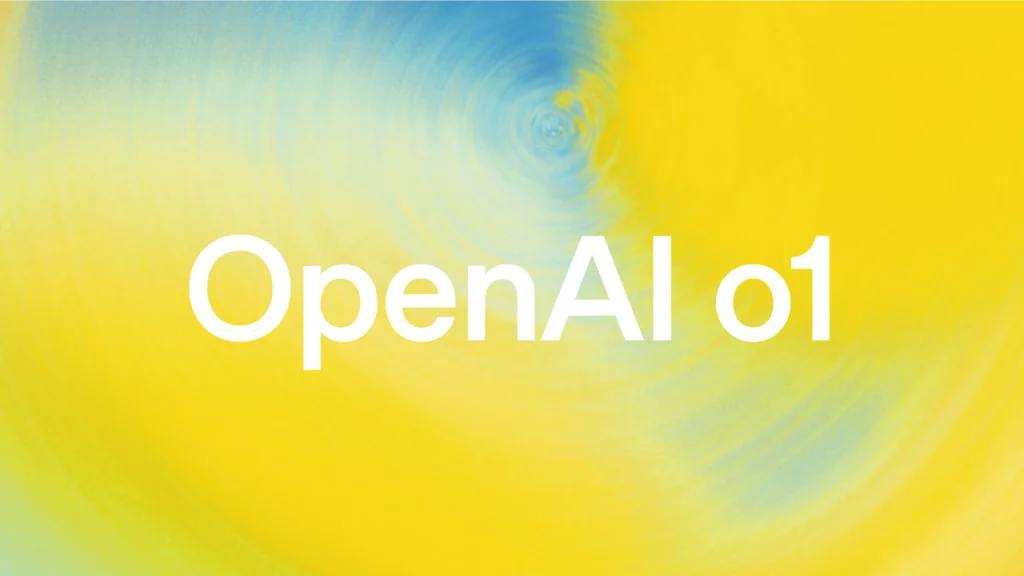
OpenAI hs recently released it’s new model o1. Each time a new model is released, researchers explore new fields where the model can be applied. On September 23rd, a paper titled <A Preliminary Study of o1 in Medicine: Are We Closer to an AI Doctor?> (Xie et al., 2024) was published, exploring the potential of AI doctors by applying o1’s logical reasoning processes to the medical field. Let’s take a closer look at AI in the medical field alongside this research paper.
AI in Medical Field
- Medical Imaging Analysis
We can use AI to diagnose and analyze medical images such as MRI, CT, and X-rays. Image processing models help accurately detect the location and size of tumors or lesions, increasing diagnostic accuracy. - Medical Natural Language Processing (NLP)
AI analyzes electronic medical records (EMR) and medical documents to extract meaningful information. It can structure textual data for easier analysis, predict drug interactions, or help create personalized treatment plans. Recently, specialized LLMs for the medical field are becoming the key to answer complex medical questions. - Biosignal Analysis
AI analyzes biosignal data like electrocardiograms (ECG) and electroencephalograms (EEG) to detect abnormal patterns. For example, real-time ECG monitoring can detect arrhythmias or myocardial infarctions early, while EEG analysis can predict epileptic seizures.
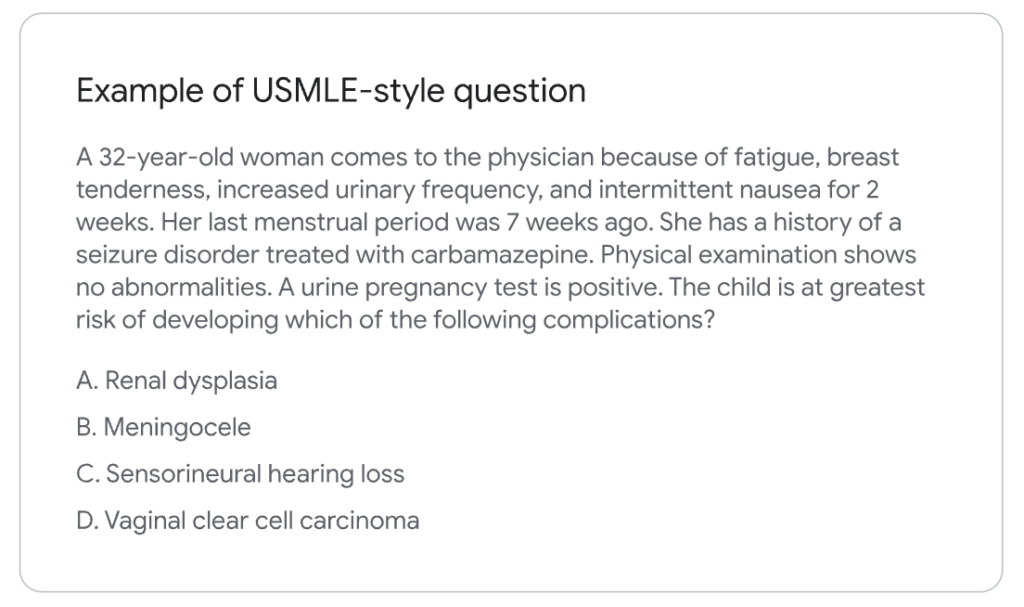
Example of Detailed Symptom Explanation and Answer Inference by AI.
Are We Getting Closer to AI Doctors?
The research also utilizes the o1 model to study the process of medical natural language processing, including symptom explanation and diagnosis. To briefly highlight the o1 model’s features, it applies Chain-of-Thought reasoning through reinforcement learning methods, leading to enhanced inference performance. In other words, o1 excels in areas where logical reasoning is necessary to reach conclusions.
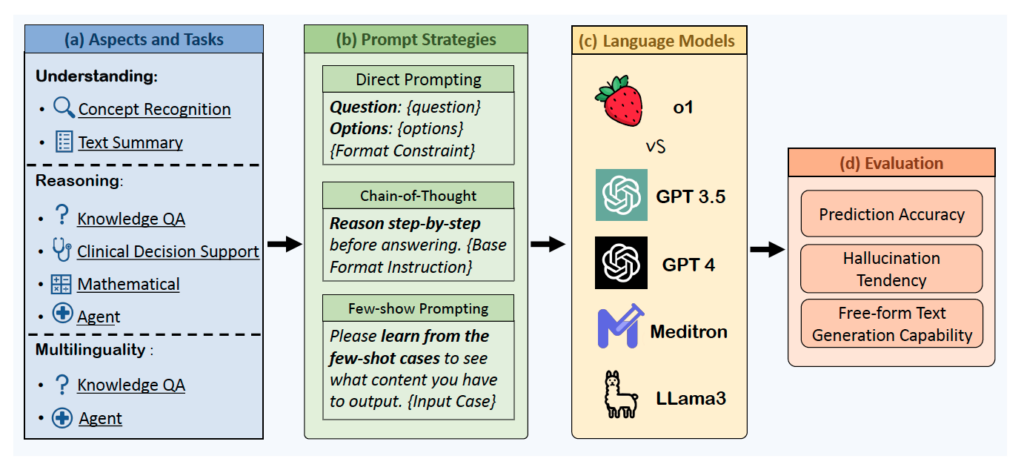
As such, the study focuses not on developing a new model but on exploring the applicability of language models in the medical field. Therefore, establishing a consistent pipeline for experiments is crucial. The researchers divide this process into the following steps: (a) deciding which tasks to handle, (b) setting up prompting strategies, (c) selecting the model, and (d) conducting evaluations.
Understanding
This task evaluates the model’s ability to understand medical concepts using its existing medical knowledge. The model is asked to extract or explain medical concepts from articles or diagnostic reports. Additionally, it may need to summarize key medical concepts concisely.
Reasoning
While understanding evaluates the knowledge the model already possesses, reasoning assesses how well the model can apply that knowledge to draw logical conclusions. The typical format involves multiple-choice questions, where the model must choose the correct answer. However, since there’s a chance the model could guess the right answer by chance, more advanced evaluations involve asking the model to make treatment suggestions based on patient information.
Multilinguality
This evaluates the model’s ability to perform tasks when the input instructions or output answers are in different languages. The XMedBench dataset includes six languages: Chinese, Arabic, Hindi, Spanish, English, and French. The model is required to answer medical questions in each of these languages.
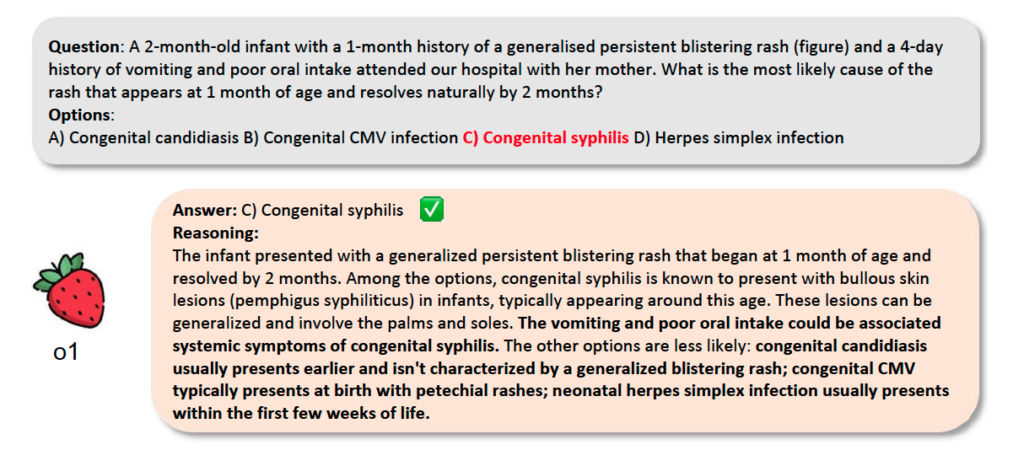
Example of o1 Model Inferring Answers on the LancetQA Dataset.
Looking at the tasks mentioned above, each dataset requires different approaches to identifying the correct answers. The evaluation metrics also differ depending on the dataset. Now, let’s compare the benchmark scores of the o1 model across various medical datasets to evaluate its performance in each task.
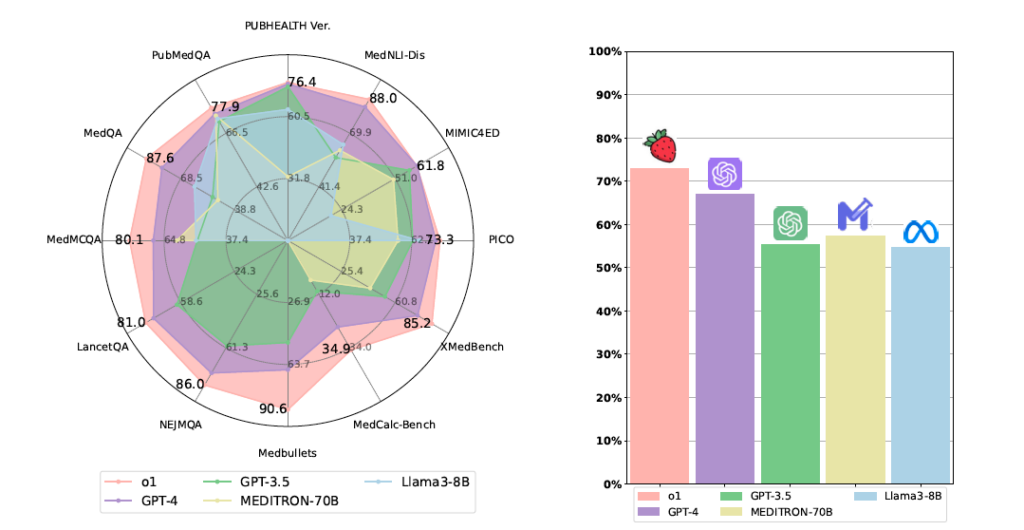
Comparison of the o1 Model with Other Language Models: Performance distribution across different datasets (left) / Average accuracy across multiple datasets (right)
Are We Getting Closer to AI Doctors?
Despite advances in language models, AI doctors face challenges, the biggest being hallucination, where the model generates false information. In medicine, explainable and trustworthy models are crucial for patient safety, and even small errors make them unsuitable for real use.
The o1 model scored 1.3% lower than GPT-4 on AlignScore, indicating more hallucinations. While o1 has improved in other areas, it remains vulnerable to this issue.
We’ve examined the o1 model’s performance in medicine. Since it focuses on reasoning rather than new learning, drastic improvements are tough. However, its strong results in medicine suggest potential in other fields. With more data or domain-specific training, o1 could become an even more valuable tool.